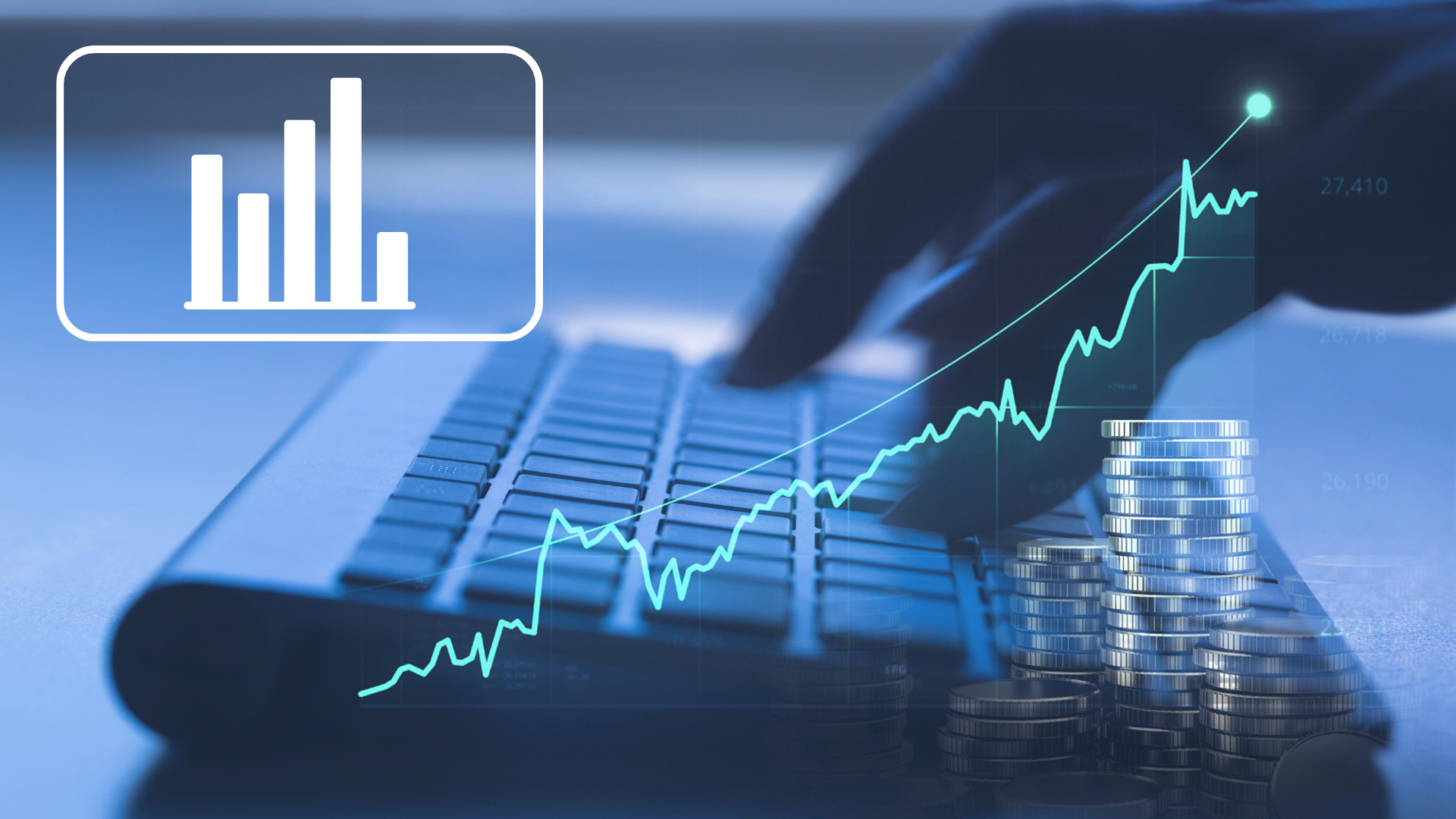
AI credit score systems represent a transformative approach to evaluating a borrower's creditworthiness. Moving beyond traditional models that depend on static variables and historical data, these systems leverage advanced machine learning algorithms to assess a wide array of data points, including non-conventional ones for prequalification purposes.
The integration of AI and predictive analytics into credit scoring is not just a trend but a necessity as the global credit scoring services market surges, expected to reach USD 54.97 billion in 2024 with a significant growth rate. This development promises to not only refine how creditworthiness is determined but also broadens the horizon for financial inclusion and precision in lending decisions.
Understanding Predictive Analytics in Credit Scoring
AI-based credit scoring signifies a pivotal shift from conventional credit evaluation methods, embracing a holistic and dynamic approach to determine a borrower's creditworthiness. Unlike traditional models that rely heavily on historical financial data and static variables, AI algorithms delve into a broader spectrum of data points, including those outside the traditional credit evaluation framework. This method encompasses:
- Data Sources Analyzed by AI:
- Traditional data: Credit history, income, debts.
- Non-traditional data: Internet browsing behavior, transaction data, social media activity, digital footprints.
- Advantages of AI in Credit Scoring:
- Inclusivity: Facilitates credit scoring for individuals with minimal or no credit history, enhancing financial inclusion.
- Accuracy: Machine learning algorithms provide more precise predictions of creditworthiness, minimizing default risks.
- Speed: AI significantly accelerates the application and scoring process compared to manual methods.
- Adaptability: AI models swiftly adjust to new data and trends, ensuring the relevance and efficacy of credit scoring criteria.
- Mechanics of Predictive Analytics:
- Data Collection: Involves gathering both traditional and non-traditional data sources.
- Model Training: Machine learning models are trained on extensive datasets to identify patterns and correlations indicative of a borrower's repayment capacity.
- Prediction: Utilizes historical data to forecast a borrower's future financial behavior and creditworthiness.
Predictive analytics, powered by AI, not only revamps the accuracy and efficiency of credit scoring but also introduces a paradigm of fairness and inclusiveness in the financial sector. By integrating alternative data sources and leveraging machine learning techniques, these models expose limitations in current evaluation methods, offering a more nuanced and comprehensive assessment of an individual's credit risk.
The Mechanics of Predictive Analytics in Enhancing Credit Scoring
The transformative power of predictive analytics in enhancing credit scoring is evident through its ability to automate the data collection and analysis process. This automation facilitates:
- Real-Time Scoring and Updates: Predictive analytics streamlines data collection, processing, and analysis stages, enabling scores to be updated in real-time or near-real-time.
- Detection of Subtle Patterns: It identifies intricate patterns and trends within vast datasets that might be overlooked by human assessors, offering a nuanced assessment of creditworthiness.
- Integration with Traditional Methods: By combining AI-based credit scoring with traditional methods, a hybrid model is created that leverages the strengths of both approaches.
Furthermore, predictive analytics exposes limitations in current credit evaluation methods. For instance, cash-flow data is highlighted as a more effective indicator of a borrower's creditworthiness compared to traditional credit scores. This insight supports the development of cash-flow scores and attributes that enhance lenders' ability to predict credit risk.
To address the opacity often associated with machine learning models, mechanisms for model explainability have been introduced. These mechanisms ensure transparency and build trust in AI-powered credit scoring systems. The evolution of model development from traditional statistical models to advanced machine learning algorithms like XGBoost underpins the sophisticated nature of predictive analytics in credit scoring. This evolution underscores the process's complexity, involving prescreening, underwriting, and portfolio risk assessment, thereby revolutionizing the approach to credit scoring.
Key Benefits of Integrating Predictive Analytics into Credit Scoring
Integrating predictive analytics into credit scoring offers a multitude of benefits, enhancing not just the accuracy but also the efficiency of credit evaluation processes. Key advantages include:
- Enhanced Accuracy and Consistency: Predictive analytics leverages vast data sets, improving the precision of credit scoring. This leads to more consistent credit decisions, reducing the likelihood of defaults.
- Cost and Time Efficiency: By automating data collection and analysis, predictive analytics significantly cuts down the time and cost involved in credit scoring processes.
- Inclusiveness and Fairness: Expanding the pool of potential borrowers, especially those with limited or no credit history, predictive analytics fosters inclusiveness and fairness in credit scoring.
Furthermore, predictive analytics revolutionizes customer experience and operational efficiency through:
- Personalized Credit Products: Offering tailored, transparent, and flexible credit solutions enhances customer satisfaction and loyalty.
- Operational Streamlining: Automating credit forecasting and risk assessment processes optimizes operational efficiency, allowing for real-time updates and early warning systems for credit risks.
Lastly, the integration of AI in predictive analytics addresses critical ethical considerations by:
- Mitigating Biases: AI models are designed to identify and correct biases in credit scoring, ensuring fair lending practices.
- Promoting Sustainable Finance: AI's accurate and transparent credit risk assessments contribute to sustainable finance by identifying financially viable and environmentally responsible lending opportunities.
By harnessing the power of predictive analytics, credit scoring becomes not only more efficient and accurate but also more inclusive and fairer, significantly enhancing the lending landscape.
Addressing Challenges and Ethical Considerations
Predictive analytics, while revolutionizing credit scoring with AI, introduces several challenges and ethical considerations that necessitate careful navigation. Key challenges include:
- Data Quality and Security: Ensuring the integrity and protection of data against breaches is paramount. This involves rigorous validation of data sources and implementing robust cybersecurity measures.
- Regulatory Compliance: Adhering to financial regulations and ethical standards, while also navigating the evolving legal landscape around big data and AI.
- Model Transparency and Fairness: Addressing potential biases in AI algorithms is critical. Regular audits and the application of ethical AI principles can help mitigate biases against protected classes like age, race, and gender.
Best practices for addressing these challenges involve:
- Data Governance: Applying strict data governance principles to manage data quality, privacy, and security.
- Ethical AI Frameworks: Implementing the five pillars of ethical AI—responsibility, transparency, predictability, auditability, and incorruptibility—to guide the development and deployment of predictive models.
- Continuous Monitoring: Regularly updating models and monitoring their performance to ensure they remain accurate and fair over time.
The integration of AI in credit scoring, while promising, requires a balanced approach that considers both the technological advancements and the ethical implications. By adopting best practices and addressing the outlined challenges, the financial industry can leverage predictive analytics to enhance credit scoring systems while ensuring fairness, transparency, and security.
So, what can we take away from this?
Throughout this discussion, it's evident that the integration of AI and predictive analytics into credit scoring heralds a significant shift in how financial worthiness is assessed. By leveraging an extensive array of both traditional and non-traditional data points, these advanced systems not only promise increased accuracy and efficiency in credit evaluations but also herald a new era of inclusivity and fairness within the financial sector. The move towards AI-driven credit scoring embodies a crucial evolution—from static, historical data reliance to a dynamic, real-time analysis that better captures an individual's financial health.
As we explore the vast potential that AI and predictive analytics have to offer, the onus lies on us to harness these technologies responsibly and equitably. For those looking to delve deeper into how AI can transform credit scoring and beyond, we invite you to book a demo and see how Qscore’s predictive analytics capabilities are reducing customer acquisition costs by 30% for global organizations.